图书介绍
STATISTICS AND DATA ANAL:YSIS FOR THE BEHAVIORAL:SCIENCES2025|PDF|Epub|mobi|kindle电子书版本百度云盘下载
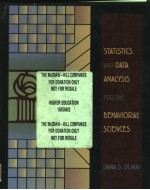
- DANA S.DUNN 著
- 出版社: MCGRAW-HILL HIGHER EDUCATION
- ISBN:
- 出版时间:2001
- 标注页数:723页
- 文件大小:65MB
- 文件页数:749页
- 主题词:
PDF下载
下载说明
STATISTICS AND DATA ANAL:YSIS FOR THE BEHAVIORAL:SCIENCESPDF格式电子书版下载
下载的文件为RAR压缩包。需要使用解压软件进行解压得到PDF格式图书。建议使用BT下载工具Free Download Manager进行下载,简称FDM(免费,没有广告,支持多平台)。本站资源全部打包为BT种子。所以需要使用专业的BT下载软件进行下载。如BitComet qBittorrent uTorrent等BT下载工具。迅雷目前由于本站不是热门资源。不推荐使用!后期资源热门了。安装了迅雷也可以迅雷进行下载!
(文件页数 要大于 标注页数,上中下等多册电子书除外)
注意:本站所有压缩包均有解压码: 点击下载压缩包解压工具
图书目录
1 INTRODUCTION: STATISTICS AND DATA ANALYSIS AS TOOLS FOR RESEARCHERS3
2 PROCESS OF RESEARCH IN PSYCHOLOGY AND RELATED FIELDS45
3 FREQUENCY DISTRIBUTIONS, GRAPHING, AND DATA DISPLAY85
4 DESCRIPTIVE STATISTICS: CENTRAL TENDENCY AND VARIABILITY133
5 STANDARD SCORES AND THE NORMAL DISTRIBUTION177
6 CORRELATION205
7 LINEAR REGRESSION241
8 PROBABILITY273
9 INFERENTIAL STATISTICS: SAMPLING DISTRIBUTIONS AND HYPOTHESIS TESTING315
10 MEAN COMPARISON Ⅰ: THE t TEST365
11 MEAN COMPARISON Ⅱ: ONE-VARIABLE ANALYSIS OF VARIANCE411
12 MEAN COMPARISON Ⅲ: TWO-VARIABLE ANALYSIS OF VARIANCE459
13 MEAN COMPARISON Ⅳ: ONE-VARIABLE REPEATED-MEASURES ANALYSIS OF VARIANCE499
14 SOME NONPARAMETRIC STATISTICS FOR CATEGORICAL AND ORDINAL DATA523
15 CONCLUSION: STATISTICS AND DATA ANALYSIS IN CONTEXT563
1 INTRODUCTION: STATISTICS AND DATA ANALYSIS AS TOOLS FOR RESEARCHERS3
DATA BOX 1.A: What Is or Are Data?5
Tools for Inference: David L.'s Problem5
College Choice6
College Choice: What Would (Did) You Do?6
Statistics Is the Science of Data, Not Mathematics8
Statistics, Data Analysis, and the Scientific Method9
Inductive and Deductive Reasoning10
Populations and Samples12
Descriptive and Inferential Statistics16
DATA BOX 1.B: Reactions to the David L. Problem18
Knowledge Base19
Discontinuous and Continuous Variables20
DATA BOX 1.c: Rounding and Continuous Variables22
Writing About Data: Overview and Agenda23
Scales of Measurement24
Nominal Scales25
Ordinal Scales26
Interval Scales27
Ratio Scales28
Writing About Scales29
Knowledge Base31
Overview of Statistical Notation31
What to Do When: Mathematical Rules of Priority34
DATA BOX 1.D: The Size of Numbers is Relative38
Mise en Place39
About Calculators39
Knowledge Base40
PROJECT EXERCISE: Avoiding Statisticophobia40
Looking Forward, Then Back41
Summary42
Key Terms42
Problems42
2 PROCESS OF RESEARCH IN PSYCHOLOGY AND RELATED FIELDS45
The Research Loop of Experimentation: An Overview of the Research Process45
Populations and Samples Revisited: The Role of Randomness48
Distinguishing Random Assignment from Random Sampling48
Some Other Randomizing Procedures50
Sampling Error52
Knowledge Base53
DATA BOX 2.A: Recognizing Randomness, Imposing Order54
Independent and Dependent Variables54
Types of Dependent Measures58
Closing or Continuing the Research Loop?60
DATA BOX 2.B: Variable Distinctions: Simple, Sublime, and All Too Easily Forgotten61
The Importance of Determining Causality61
DATA BOX 2.C: The “Hot Hand in Basketball” and the Misrepresentation of Randomness62
Operational Definitions in Behavioral Research63
Writing Operational Definitions64
Knowledge Base64
Reliability and Validity65
Reliability66
Validity67
Knowledge Base69
Research Designs70
Correlational Research70
Experiments72
Quasi-experiments74
DATA BOX 2.D: Quasi-experimentation in Action: What to Do Without Random Assignment or a Control Group75
Knowledge Base76
PROJECT EXERCISE: Using a Random Numbers Table77
Looking Forward, Then Back81
Summary81
Key Terms82
Problems82
3 FREQUENCY DISTRIBUTIONS, GRAPHING, AND DATA DISPLAY85
What is a Frequency Distribution?87
DATA BOX 3.A: Dispositional Optimism and Health: A Lot About the LOT88
Proportions and Percentages90
Grouping Frequency Distributions92
True Limits and Frequency Distributions95
Knowledge Base96
Graphing Frequency Distributions97
Bar Graphs98
Histograms99
Frequency Polygons100
Misrepresenting Relationships: Biased or Misleading Graphs102
New Alternatives for Graphing Data: Exploratory Data Analysis104
Stem and Leaf Diagrams105
DATA BOX 3.B: Biased Graphical Display—Appearances Can Be Deceiving106
Tukey's Tallies108
Knowledge Base109
Envisioning the Shape of Distributions111
DATA BOX 3.c: Kurtosis, or What's the Point Spread?113
DATA BOX 3.D: Elegant Information—Napoleon's Ill-fated March to Moscow114
Percentiles and Percentile Ranks115
Cumulative Frequency116
Cumulative Percentage117
Calculating Percentile Rank118
Reversing the Process: Finding Scores from Percentile Ranks119
Exploring Data: Calculating the Middle Percentiles and Quartiles120
Writing About Percentiles122
Knowledge Base123
Constructing Tables and Graphs123
Less is More: Avoiding Chartjunk and Tableclutter, and Other Suggestions124
American Psychological Association (APA) Style Guidelines for Data Display125
PROJECT EXERCISE: Discussing the Benefits of Accurate but Persuasive Data Display126
Looking Forward, Then Back127
Summary128
Key Terms129
Problems129
4 DESCRIPTIVE STATISTICS: CENTRAL TENDENCY AND VARIABILITY133
Why Represent Data By Central Tendency134
The Mean: The Behavioral Scientist's Statistic of Choice136
DATA BOX 4.A: How Many Are There? And Where Did They Come138
From? Proper Use of N and n138
Calculating Means from Ungrouped and Grouped Data138
Caveat Emptor: Sensitivity to Extreme Scores140
Weighted Means: An Approach for Determining Averages of Different-Sized Groups142
DATA BOX 4.B: Self-Judgment Under Uncertainty—Being Average is Sometimes OK143
The Median144
The Mode145
The Utility of Central Tendency147
Shapes of Distributions and Central Tendency147
When to Use Which Measure of Central Tendency148
Writing About Central Tendency149
Knowledge Base150
Understanding Variability151
The Range153
The Interquartile and the Semi-Interquartile Range153
Variance and Standard Deviation155
Sample Variance and Standard Deviation157
Homogeneity and Heterogeneity: Understanding the Standard Deviations of Different Distributions159
Calculating Variance and Standard Deviation from a Data Array160
Population Variance and Standard Deviation161
Looking Ahead: Biased and Unbiased Estimators of Variance and Standard Deviation162
DATA BOX 4.c: Avoid Computation Frustration: Get to Know Your Calculator165
Knowledge Base165
Factors Affecting Variability166
Writing About Range, Variance, and Standard Deviation168
DATA BOX 4.D: Sample Size and Variability—The Hospital Problem169
PROJECT EXERCISE: Proving the Least Squares Principle for the Mean170
Looking Forward, Then Back171
Summary172
Key Terms173
Problems173
5 STANDARD SCORES AND THE NORMAL DISTRIBUTION177
DATA BOX 5.A: Social Comparison Among Behavioral and Natural Scientists: How Many Peers Review Research Before Publication?179
DATA BOX 5.B: Explaining the Decline in SAT Scores: Lay Versus Statistical Accounts180
Why Standardize Measures?181
The z Score: A Conceptual Introduction182
Formulas for Calculating z Scores185
The Standard Normal Distribution186
Standard Deviation Revisited: The Area Under the Normal Curve187
Application: Comparing Performance on More than One Measure188
Knowledge Base189
Working with z Scores and the Normal Distribution190
Finding Percentile Ranks with z Scores191
Further Examples of Using z Scores to Identify Areas Under the Normal Curve192
DATA BOX 5.C: Intelligence, Standardized IQ Scores, and the Normal Distribution194
A Further Transformed Score: The T Score196
Writing About Standard Scores and the Normal Distribution197
Knowledge Base198
Looking Ahead: Probability, z Scores, and the Normal Distribution198
PROJECT EXERCISE: Understanding the Recentering of Scholastic Aptitude Test Scores199
Looking Forward, Then Back201
Summary202
Key Terms202
Problems202
6 CORRELATION205
Association, Causation, and Measurement206
Galton, Pearson, and the Index of Correlation207
A Brief But Essential Aside: Correlation Does Not Imply Causation207
The Pearson Correlation Coefficient209
Conceptual Definition of the Pearson r209
DATA BOX 6.A: Mood as Misbegotten: Correlating Predictors with Mood States213
Calculating the Pearson r216
Interpreting Correlation221
Magnitude of r222
Coefficients of Determination and Nondetermination222
Factors Influencing r224
Writing About Correlational Relationships226
Knowledge Base227
Correlation as Consistency and Reliability228
DATA BOX 6.B: Personality, Cross-Situational Consistency, and Correlation228
Other Types of Reliability Defined229
A Brief Word About Validity229
DATA BOX 6.c: Examining a Correlation Matrix: A Start for Research230
What to Do When: A Brief, Conceptual Guide to Other Measures of Association231
DATA BOX 6.D: Perceived Importance of Scientific Topics and Evaluation Bias232
PROJECT EXERCISE: Identifying Predictors of Your Mood233
Looking Forward, Then Back237
Summary237
Key Terms238
Problems238
7 LINEAR REGRESSION241
Simple Linear Regression242
The z Score Approach to Regression242
Computational Approaches to Regression243
The Method of Least Squares for Regression245
Knowledge Base249
DATA BOX 7.A: Predicting Academic Success250
Residual Variation and the Standard Error of Estimate251
DATA BOX 7.B: The Clinical and the Statistical: Intuition Versus Prediction253
Assumptions Underlying the Standard Error of Estimate253
Partitioning Variance: Explained and Unexplained Variation256
A Reprise for the Coefficients of Determination and Nondetermination257
Proper Use of Regression: A Brief Recap258
Knowledge Base258
Regression to the Mean259
DATA BOX 7.c: Reinforcement, Punishment, or Regression Toward the Mean?260
Regression as a Research Tool261
Other Applications of Regression in the Behavioral Sciences262
Writing About Regression Results263
Multivariate Regression: A Conceptual Overview263
PROJECT EXERCISE: Perceiving Risk and Judging the Frequency of Deaths264
Looking Forward, Then Back268
Summary268
Key Terms269
Problems269
8 PROBABILITY273
The Gambler's Fallacy or Randomness Revisited275
Probability: A Theory of Outcomes277
Classical Probability Theory277
DATA BOX B.A: “I Once Knew a Man Who&”: Beware Man-Who Statistics278
Probability's Relationship to Proportion and Percentage281
DATA BOX 8.B: Classical Probability and Classic Probability Examples282
Probabilities Can Be Obtained from Frequency Distributions283
Knowledge Base283
DATA BOX 8.c: A Short History of Probability284
Calculating Probabilities Using the Rules for Probability285
The Addition Rule for Mutually Exclusive and Nonmutually Exclusive Events285
The Multiplication Rule for Independent and Conditional Probabilities287
DATA BOX 8.D: Conjunction Fallacies: Is Linda a Bank Teller or a Feminist Bank Teller?288
Multiplication Rule for Dependent Events293
Knowledge Base293
Using Probabilities with the Standard Normal Distribution: z Scores Revisited294
Determining Probabilities with the Binomial Distribution: An Overview299
Working with the Binomial Distribution300
Approximating the Standard Normal Distribution with the Binomial Distribution301
DATA BOX 8.E: Control, Probability, and When the Stakes Are High304
Knowledge Base305
p Values: A Brief Introduction305
Writing About Probability306
PROJECT EXERCISE: Flipping Coins and the Binomial Distribution307
Looking Forward, Then Back310
Summary310
Key Terms311
Problems311
9 INFERENTIAL STATISTICS: SAMPLING DISTRIBUTIONS AND HYPOTHESIS TESTING315
Samples, Population, and Hypotheses: Links to Estimation and Experimentation316
Point Estimation317
Statistical Inference and Hypothesis Testing318
The Distribution of Sample Means319
Expected Value and Standard Error320
The Central Limit Theorem322
Law of Large Numbers Redux322
DATA BOX 9.A: The Law of Small Numbers Revisited323
Standard Error and Sampling Error in Depth324
Estimating the Standard Error of the Mean324
Standard Error of the Mean: A Concrete Example Using Population Parameters326
Defining Confidence Intervals Using the Standard Error of the Mean327
DATA BOX 9.B: Standard Error as an Index of Stability and Reliability of Means328
Knowledge Base329
DATA BOX 9.C: Representing Standard Error Graphically330
Asking and Testing Focused Questions: Conceptual Rationale for Hypotheses331
DATA BOX 9.D: What Constitutes a Good Hypothesis?332
Directional and Nondirectional Hypotheses333
The Null and the Experimental Hypothesis333
Statistical Significance: A Concrete Account336
DATA BOX 9.E: Distinguishing Between Statistical and Practical Significance337
Critical Values: Establishing Criteria for Rejecting the Null Hypothesis338
One- and Two-Tailed Tests340
Degrees of Freedom341
DATA BOX 9.F: When the Null Hypothesis is Rejected—Evaluating Results with the MAGIC Criteria342
Knowledge Base343
Single Sample Hypothesis Testing: The z Test and the Significance of r343
What Is the Probability a Sample Is from One Population or Another?344
Is One Sample Different from a Known Population?345
When Is a Correlation Significant?347
Inferential Errors Types Ⅰ and Ⅱ349
Statistical Power and Effect Size351
Effect Size354
Writing About Hypotheses and the Results of Statistical Tests355
Knowledge Base357
PROJECT EXERCISE: Thinking About Statistical Significance in the Behavioral Science Literature357
Looking Forward, Then Back360
Summary360
Key Terms362
Problems362
10 MEAN COMPARISON I: THE t TEST365
Recapitulation: Why Compare Means?367
The Relationship Between the t and the z Distributions368
The t Distribution368
Assumptions Underlying the t Test369
DATA BOX 10.A: Some Statistical History: Who was “A Student”?371
Hypothesis Testing with t: One-Sample Case372
Confidence Intervals for the One-Sample t Test375
DATA BOX 10.B: The Absolute Value of t376
Power Issues and the One-Sample t Test377
Knowledge Base377
Hypothesis Testing with Two Independent Samples378
Standard Error Revised: Estimating the Standard Error of the Difference Between Means379
Comparing Means: A Conceptual Model and an Aside for Future Statistical Tests383
The t Test for Independent Groups384
DATA BOX 10.C: Language and Reporting Results, or (Too) Great Expectations388
Effect Size and the t Test388
Characterizing the Degree of Association Between the Independent Variable and the Dependent Measure389
DATA BOX 10.D: Small Effects Can Be Impressive Too390
Knowledge Base392
Hypothesis Testing with Correlated Research Designs393
The Statistical Advantage of Correlated Groups Designs: Reducing Error Variance395
The t Test for Correlated Groups396
Calculating Effect Size for Correlated Research Designs399
A Brief Overview of Power Analysis: Thinking More Critically About Research and Data Analysis400
Knowledge Base402
PROJECT EXERCISE: Planning for Data Analysis: Developing a Before and After Data Collection Analysis Plan402
Looking Forward, Then Back405
Summary405
Key Terms406
Problems406
11 MEAN COMPARISON Ⅱ: ONE-VARIABLE ANALYSIS OF VARIANCE411
Overview of the Analysis of Variance413
Describing the F Distribution417
Comparing the ANOVA to the t Test: Shared Characteristics and Assumptions418
Problematic Probabilities: Multiple t Tests and the Risk of Type I Error420
DATA BOX 11.A: R. A. Fischer: Statistical Genius and Vituperative Visionary422
How is the ANOVA Distinct from Prior Statistical Tests? Some Advantages423
Omnibus Test: Comparing More than Two Means Simultaneously423
DATA BOX 1 1.B: Linguistically Between a Rock and Among Hard Places424
Experimentwise Error: Protecting Against Type I Error424
Causality and Complexity425
Knowledge Base426
One-Factor Analysis of Variance426
Identifying Statistical Hypotheses for the ANOVA427
Some Notes on Notation and the ANOVA's Steps429
DATA BOX 1 1.C: Yet Another Point of View on Variance: The General Linear Model431
One-Way ANOVA from Start to Finish: An Example with Data431
Post Hoc Comparisons of Means: Exploring Relations in the “Big, Dumb F”439
Tukey's Honestly Significant Difference Test440
Effect Size for the F Ratio442
Estimating the Degree of Association Between the Independent Variable and the Dependent Measure443
DATA BOX 11.D: A Variance Paradox—Explaining Variance Due to Skill or Baseball is Life444
Writing About the Results of a One-Way ANOVA445
Knowledge Base446
An Alternative Strategy for Comparing Means: A Brief Introduction to Contrast Analysis447
PROJECT EXERCISE: Writing and Exchanging Letters About the ANOVA451
Looking Forward, Then Back452
Summary453
Key Terms454
Problems454
12 MEAN COMPARISON Ⅲ: TWO-VARIABLE ANALYSIS OF VARIANCE459
Overview of Complex Research Designs: Life Beyond Manipulating One Variable460
Two-Factor Analysis of Variance461
DATA BOX 12.A: Thinking Factorially463
Reading Main Effects and the Concept of Interaction465
Statistical Assumptions of the Two-Factor ANOVA469
Hypotheses, Notation, and Steps for Performing for the Two-Way ANOVA469
DATA BOX 12.B: Interpretation Qualification: Interactions Supercede Main Effects471
The Effects of Anxiety and Ordinal Position on Affiliation: A Detailed Example of a Two-Way ANOVA475
Knowledge Base475
DATA BOX 12.C: The General Linear Model for the Two-Way ANOVA476
Effect Size486
Estimated Omega-Squared (w2) for the Two-Way ANOVA487
Writing About the Results of a Two-Way ANOVA488
Coda: Beyond 2 × 2 Designs489
Knowledge Base490
PROJECT EXERCISE: More on Interpreting Interaction—Mean Polish and Displaying Residuals490
Looking Forward, Then Back495
Summary495
Key Terms495
Problems496
13 MEAN COMPARISION Ⅳ: ONE-VARIABLE REPEATED-MEASURES ANALYSIS OF VARIANCE499
One-Factor Repeated-Measures ANOVA501
Statistical Assumptions of the One-Way Repeated-Measures ANOVA502
Hypothesis, Notation, and Steps for Performing the One-Variable Repeated-Measures ANOVA503
DATA BOX 13.A: Cell Size Matters, But Keep the Cell Sizes Equal, Too508
Tukey's HSD Revisited510
Effect Size and the Degree of Association Between the Independent Variable and Dependent Measure511
Writing About the Results of a One-Way Repeated-Measures Design512
Knowledge Base513
DATA BOX 13.B: Improved Methodology Leads to Improved Analysis—Latin Square Designs514
Mixed Design ANOVA: A Brief Conceptual Overview of Between-Within Research Design515
PROJECT EXERCISE: Repeated-Measures Designs: Awareness of Threats to Validity and Inference516
Looking Forward, Then Back518
Summary518
Key Terms519
Problems519
14 SOME NONPARAMETRIC STATISTICS FOR CATEGORICAL AND ORDINAL DATA523
How Do Nonparametric Tests Differ from Parametric Tests?525
Advantages of Using Nonparametric Statistical Tests Over Parametric Tests526
Choosing to Use a Nonparametric Test: A Guide for the Perplexed527
DATA BOX 14.A: The Nonparametric Bible for the Behavioral Sciences: Siegel and Castellan (1988)528
The Chi-Square (x2) Test for Categorical Data528
Statistical Assumptions of the Chi-Square529
The Chi-Square Test for One-Variable: Goodness-of-Fit529
The Chi-Square Test of Independence of Categorical Variables534
DATA BOX 14.B: A Chi-Square Test for Independence Shortcut for 2 × 2 Tables538
Supporting Statistics for the Chi-Square Test of Independence: Phi(?) and Cramer's V538
Writing About the Result of a Chi-Square Test for Independence539
DATA BOX 14.C: Research Using the Chi-Square Test to Analyze Data540
Knowledge Base541
Ordinal Data: A Brief Overview541
The Mann-Whitney U Test541
DATA BOX 14.D: Handling Tied Ranks in Ordinal Data544
Mann-Whitney U Test for Larger (Ns > 20) Samples: A Normal Approximation of the U Distribution546
Writing About the Results of the Mann-Whitney U Test547
The Wilcoxon Matched-Pairs Signed-Ranks Test547
DATA BOX 14.E: Even Null Results Must Be Written Up and Reported550
Writing About the Results of the Wilcoxon (T) Test551
The Spearman Rank Order Correlation Coefficient551
Writing About the Results of a Spearman rs Test554
Knowledge Base554
DATA BOX 14.F: Research Using An Ordinal Test to Analyze Data555
PROJECT EXERCISE: Survey Says—Using Nonparametric Tests on Data556
Looking Forward, Then Back558
Summary558
Key Terms559
Problems559
15 CONCLUSION: STATISTICS AND DATA ANALYSIS IN CONTEXT563
The Fuss Over Null Hypothesis Significance Tests564
Panel Recommendations: Wisdom from the APA Task Force on Statistical Inference565
Knowledge Base567
Statistics as Avoidable Ideology567
Reprise: Right Answers Are Fine, but Interpretation Matters More568
Linking Analysis to Research569
Do Something: Collect Some Data, Run a Study, Get Involved569
Knowing When to Say When: Seeking Statistical Help in the Future570
DATA BOX 15.A: Statistical Heuristics and Improving Inductive Reasoning571
Data Analysis with Computers: The Tools Perspective Revisited572
Knowledge Base573
Thinking Like a Behavioral Scientist: Educational, Social, and Ethical Implications of Statistics and Data Analysis573
DATA BOX 15.B: Recurring Problems with Fraudulent, False, or Misleading Data Analysis: The Dracula Effect576
Conclusion578
PROJECT EXERCISE: A Checklist for Reviewing Published Research or Planning a Study578
Looking Forward, Then Back580
Summary580
Key Terms581
Problems581
热门推荐
- 3089424.html
- 1757848.html
- 641228.html
- 1723053.html
- 1923257.html
- 2553735.html
- 936905.html
- 351078.html
- 3095769.html
- 1781440.html
- http://www.ickdjs.cc/book_1354414.html
- http://www.ickdjs.cc/book_1597090.html
- http://www.ickdjs.cc/book_3867488.html
- http://www.ickdjs.cc/book_3343159.html
- http://www.ickdjs.cc/book_2357820.html
- http://www.ickdjs.cc/book_1924872.html
- http://www.ickdjs.cc/book_622082.html
- http://www.ickdjs.cc/book_1916027.html
- http://www.ickdjs.cc/book_3779675.html
- http://www.ickdjs.cc/book_2325290.html